
Clark Quinn
We often hear learning businesses say they want to better engage their learners. But what is engagement, and how does it work?
In this episode, Leading Learning Podcast co-host Jeff Cobb digs into those questions with Clark Quinn, executive director of Quinnovation and author of Make It Meaningful: Taking Learning Design from Instructional to Transformational.
Jeff and Clark talk about engagement and motivation; learners’ cognitive, affective, and conative involvement; and how the cognitive aspect gets more than its fair share of emphasis. They also talk about the role of emotion in learning, about practice and feedback, and about how the ability to make better decisions is ultimately the desired outcome of most learning experiences.
To tune in, listen below. To make sure you catch all future episodes, be sure to subscribe via RSS, Apple Podcasts, Spotify, Stitcher Radio, iHeartRadio, PodBean, or any podcatcher service you may use (e.g., Overcast). And, if you like the podcast, be sure to give it a tweet.
Listen to the Show
Access the Transcript
Download a PDF transcript of this episode’s audio.
Read the Show Notes
Clark Quinn: [00:00:00] There’s a large focus on the cognitive side, but there’s much less focus on the engagement side. And yet we have evidence that it matters a lot, particularly your intent to commit to learn.
Celisa Steele: [00:00:17] I’m Celisa Steele.
Jeff Cobb: [00:00:18] I’m Jeff Cobb, and this is the Leading Learning Podcast.
Celisa Steele: [00:00:26] We often hear learning businesses describe a desire to better engage their learners. But what is engagement, and how does one engage learners? This episode, number 405, digs into those questions with Clark Quinn. Clark wears many hats. He’s executive director of Quinnovation, chief learning strategist for Upside Learning, co-director of the Learning Development Accelerator, and science advisor to elevator9. Jeff and Clark talk about applying learning science to how learning experiences are designed, developed, and delivered—and how there’s still more work to do to make sure products and services are well aligned with learning science. They talk about engagement and motivation; about learners’ cognitive, affective, and conative involvement; and about how the cognitive aspect has gotten more than its share of emphasis over time. They talk about the role of emotion in learning, about practice and feedback, and about how, broadly speaking, the ability to make better decisions is the desired outcome of most learning experiences. Jeff and Clark spoke in March 2024.
Applying Learning Science to Achieve Better Outcomes
Jeff Cobb: [00:01:47] Can you say a little bit more, for listeners who may be less familiar with you, about the specific work that you do?
Clark Quinn: [00:01:55] The underlying theme, I would say, is that focus on applying learning science in ways that lead to better outcomes for individuals, for organizations, and hopefully for society as a whole.
Jeff Cobb: [00:02:11] That certainly jibes with how I think about you, how I’ve interpreted your work. It seems to be very, very rooted in this desire to see learning science become much more integral to how we design and develop and deliver learning experiences. So I thought it would be interesting to get your thoughts right here at the very outset of our conversation on how are we doing on that? How much progress have you seen over your years of working in learning and development? Are we applying learning science more/better now, or are there areas where you still see significant room for improvement?
Clark Quinn: [00:02:46] The short answer is yes. But I am seeing increased attention to learning science. There are more books about it; there are more talks about it; people are caring more about it. And yet the flip side is we’re still not anywhere near where we could and should be as a whole. There are pockets of great innovation and application, and there are places where they’re still going, “Wait, if it looks like school, it’s learning, right? We’ll present knowledge, test knowledge, and we’re done.” No, but I get where they came from. We’re just trying to move the needle on that.
Jeff Cobb: [00:03:26] But you’re still out there fighting the good fight, I know, along with a number of other good people who we’ve also had on the podcast—folks like Will Thalheimer, Julie Dirksen, and those types.
What Is Engagement, and What Does Learning Science Say About It?
Jeff Cobb: [00:03:35] Now, part of the reason you’re concerned with learning science is to be able to use it to create more engaging learning experiences, and that concept of engagement is something I’ve seen you devote a lot of attention to. It was really front and center in the most recent of your books, Make It Meaningful. I know I hear all the time from listeners, from clients that we work with, that what they want to do is create more engaging learning experiences. So let’s take a look at that. What does learning science tell us about how to achieve that goal, how to have those engaging learning experiences? And it might be helpful to preface that with what engagement is in the context of learning because people say “engaging,” “we want engagement,” and you’re never quite sure what they mean by that.
Clark Quinn: [00:04:21] Thanks for the opportunity because I was going to say I want to take a step back. From the cognitive science perspective, cognitive science was an interdisciplinary approach to thinking. When they realized philosophers, neuroscientists, linguists, and psychologists were all talking about thinking, and they weren’t talking to one another, they created the field cognitive science. And even cognitive science recognizes that cognition is only one aspect of how we engage. They talk about what we know. But then there’s also our intent to apply it, and they called that the conative aspect. And then there was the affective, which is who you are, which is largely immutable. But that conative is the intent to learn, and they similarly recognized, about a decade later, that there were a bunch of people looking at learning—cognitive scientists, educational psychologists, educators, and instructional designers—and they thought, “We need a way to come together.”
Clark Quinn: [00:05:15] And, as they pulled it together, they recognized that there’s a large focus on the cognitive side, but there’s much less focus on the engagement side. And yet we have evidence that it matters a lot, particularly your intent to commit to learn. We can give people learning, or—to paraphrase Dorothy Parker—you can lead a learner to learning, but you can’t make them think. But, if we open them up and help them know “I do need this, and I don’t know it already, and this experience will change that,” you’re going to get their willingness to at least beginning to engage, and then you have to deliver on that promise by ensuring that there’s the right level of challenge. Everything they’re doing is obviously of meaning to that trajectory, of moving from not knowing this to knowing this. Caring about them along the way and being supportive of their greatest success, you obtain and maintain that intent to learn.
Clark Quinn: [00:06:15] You have to get the learning elements right. You have to have the right cognitive things, but you also have to have the right emotional things. I use the word emotional as a shorthand, but it’s a shorthand for your motivation to learn, keeping anxiety low. It turns out a little bit of anxiety is conducive to learning, but too much will extinguish it, and learning itself is typically anxiety-inducing enough; you don’t need to add any more. So we make it safe to learn. We keep people’s motivation up. We build their confidence. And these are all not technically emotions yet a side that the cognitive side doesn’t tend to address and yet are important for learning. My previous book had been on the learning science, and I wanted to complement to talk about the engagement side, and that’s why I wrote the Make It Meaningful book and tried to summarize what’s known and apply it in a practical way so people can generate the engaging experiences you’re talking about.
The Role of Emotions in Motivation and Learning
Jeff Cobb: [00:07:14] You referenced emotion there, and I think we do tend to, if not completely overlook…we just don’t emphasize enough the role of emotion in learning. I think, to a large extent, people who are designing learning experiences, even if they are thinking about emotion, they’re just not really sure how do I connect with a learner emotionally? How do we do that better? You talk a lot about stories. I know stories are important. Say more about how we are able to get that emotional connection with learners.
Clark Quinn: [00:07:44] I break it up into two parts. One is the initial hook, to use a fishing metaphor—and I’m not a fishing person, so I may bugger this up. But we need to initially hook their interest, and then, again, we need to deliver on that promise, which the metaphor is landing them. You hook them, but then you’ve got to land them still. And I think the important component is the “What’s in it for me?” We have to help them understand why they should care. Too often we go, “This is the learning you have to have,” and we never address why. Even somebody who knows better, when I was writing about this, commented on my blog post. I said, “Well, tell me about your syllabus,” and he sent it to me, and I look at it and go, “Nowhere do you tell them why they should do this.” He goes, “You’re right. Thank you for giving me lots more work to do.”
Clark Quinn: [00:08:31] But that’s just an element we neglect. And really, of the instructional design theorists, for instance, John Keller is really the only one who’s talked about motivation. So he has his ARCS Model: attention, relevance, confidence, and satisfaction, which are all great elements. It gives prescriptions, but it’s not quite, in my mind, the full picture. And so that’s what we’ve tried to pull together. How does surprise play a role in this, and how do we do this? But, at core, I think it’s about just helping them go, “I do need this. I need this.” It’s that “What’s in it for me? And there’s something I could be doing that I’m not doing now that matters.”
Jeff Cobb: [00:09:10] How do game elements fit into this? Because, obviously, people can get really charged up in game-type environments, and there’s always a lot of buzz out there about “Let’s build gamification into our learning” and that sort of thing. How does it connect into emotion? How does it connect into engagement?
Clark Quinn: [00:09:26] It’s interesting. We had Mark Oehlert on the LDA [Learning Development Accelerator] earlier this week talking about Think Like an Anthropologist, and he pointed to Raph Koster. Raph Koster is a game designer who wrote a book called A Theory of Fun, and in it he claims what makes games fun is learning, which actually, I think, is quite relevant. My very first book was on how do you design games for learning. And, in general, I want to separate out extrinsic motivation from intrinsic motivation. Actually, in the most recent book, I point to Deci and Ryan’s Self-Determination Theory that my partner in the LDA, Matt Richter, pointed me to. They talk about that distinction. There’s guilt: “Oh, I should do this. Or we just add points, and, oh, well, I’m getting this extrinsic motivation. I think there’s evidence that can be damaging. If you do it wrong, it can extinguish interest—or it only works for the 10 percent of people who are getting high scores, and, the rest of the people, it turns them off. Unless you have a highly competitive group, which can happen.
Clark Quinn: [00:10:27] But I’m much more interested in finding the intrinsic interest. Why should I care? And it’s not just points, but what is it about the inherent nature of this that is meaningful to me? What games do really well—you don’t play every game that’s out there. There’s a certain genre or certain games that work for you, and they have been chosen because they work, because they tap into some story you care about. And then games are wonderfully tuned. They spend buckets of money to fine-tune. It was Will Wright, one of the game designers, a game designer—he did SimCity and The Sims. He’s the one who said, “Look, building the model is one-tenth of the work. Tuning it is nine-tenths of the work,” and it’s all that work that they can spend to get the right ratio of rewards to actions—whether it’s the randomness of the loot you collect or how you advance—and integrating all this into a coherent whole can really play a role. That’s great when you’re designing a game. And I have designed games for learning.
Clark Quinn: [00:11:36] But even we can do that in non-game learning experiences just by making better contexts. It turns out learning science tells us we learn better in context than on abstract problems. What we can do from the emotional side is make that context compelling. Instead of just working on another patient, why not save the ambassador’s daughter or the rebel leader’s daughter? It’s more compelling. We’re not just making another deal for a little bit more commission. We’re doing the deal that’s going to save the company from going under. We have ways to ramp up this exaggeration. If you look at movies and TV, they ramp up exaggeration—real life to something that’s one level above but still compelling. And we can do that, and we should, because what we find is that it accelerates the time to learning and makes it more effective. It sticks better when we do this right. And that’s, I think, powerful reasons to do this.
Partner with Tagoras
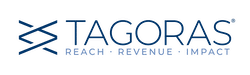
Celisa Steele: [00:12:35] At Tagoras, we partner with professional and trade associations, continuing education units, training firms, and other learning businesses to help them to understand market realities and potential, to connect better with existing customers and find new ones, and to make smart investment decisions around product development and portfolio management. Drawing on our expertise in lifelong learning, market assessment, and strategy formulation, we can help you achieve greater reach, revenue, and impact. Learn more at tagoras.com/more.
What Learning Science Tells Us About Practice and Feedback
Jeff Cobb: [00:13:14] When you’re engaged in a game, you’re typically practicing and getting feedback. You may not be thinking about it that way, but that’s what you’re doing. I think most people who develop learning experiences do have some understanding that providing for practice and giving feedback are important for effective learning. But I think it trips a lot of people up. Either they make it more complicated than it needs to be, or they provide practice and feedback—and you mentioned meaning earlier and things being meaningful—that really aren’t very meaningful to the learner. It just feels like you’re going through the motions. So what does learning science tell us about how to do practice and feedback well?
Clark Quinn: [00:13:55] Contextualized practice matters. We want good story settings, but we should make them ones that the learner recognizes are relevant. And the challenge needs to be pitched at the right level. We learn that from games—too simple, and they’re boring. We can also learn that from Csikszentmihalyi’s Flow, and it happens to match really nicely with Vygotsky’s notion of Zone of Proximal Development. Too simple, no learning happens, and it’s boring. Too complex, it’s too frustrating, and no learning happens. And in between is the zone. And that changes as you develop your abilities. You need more challenges. You need the right level of challenge and the right context, a good story that is a real application of that skill and yet one that the learner recognizes. It doesn’t have to be set in the real world; it can be set in a fantastic world. And there are reasons, particularly in the case of needing far transfer, where that may actually facilitate your ability to abstract the underlying principles and then remap. Because from learning science, we have two goals: retention over time until it’s needed and transfer to all appropriate, no inappropriate situations.
Clark Quinn: [00:14:58] By practicing across contexts—the multiple practice spaced over time supports retention, which is why games have you do it again and again. You face this opponent, that opponent, that opponent, and, to Raph Koster’s point, at the end is a test. At the end of a level is a test—it’s the boss. You’ve practiced these maneuvers, and now you really have to put them all together to get past the boss. That’s a test. It’s learning. But then feedback. People are beginning to recognize that the best feedback comes in the form of consequences of your actions in some way. It may be your boss saying, “That was a bad decision.” It may be your customer saying, “Nope, that didn’t work.” It may be your patient dying. Whatever it is. The didactic feedback was the wrong choice because this should come after the experience.
Clark Quinn: [00:15:45] You don’t want to break the experience and stop and say, “No, that’s the wrong answer. Okay, now continue.” Particularly as you gain expertise, your feedback can be delayed. But games, as you point out, really are multiple-choice questions. Do I shoot this opponent versus that opponent? Do I run away? These are all choices you make. And then the consequence of those actions plays out. You may die, and you have to learn why. Better try not running away this time because it didn’t work. Whatever it is. You’re having choices and feedback, and they are tuned to be at the right level of challenge and have the right level of randomness and the right level of rewards. We need to be thinking about that from a learning perspective as well. What are the right decisions? What are the consequences of that? How do they tie together? Really good learning designs are mini scenarios, and, if you string them together, you get branching scenarios, or you can have a full simulation-driven experience underneath that’s really a game. But, whatever it is, it’s the core thing.
Clark Quinn: [00:16:45] By the way, editorial comment. This is what AI is not yet going to be able to do, and perhaps for the foreseeable future, is actually determine what are the right decisions we need to embed in sequence at the right level and a variety of contexts to span. And then AI can help us write really compelling scenarios. But, as yet, I think the role of designers is going to be embedding the right decisions in a meaningful context and choosing the right sequence of consequences and feedback. And we know what makes good feedback. Learning science tells us, in addition to practice being contextual and challenging and spaced and whatever, feedback needs to be minimal, based on the behavior, not the person, specifically referred to the underlying mental model that tells you how the world works, and should guide your decisions. All that stuff can happen. But the world should tell it to you first, and then, after the experience, you can provide the didactic feedback.
Making Better Decisions: The Ultimate Goal of Most Learning
Jeff Cobb: [00:17:45] You used a word in there a few times that has jumped out to me in your writing, and that’s decisions—making decisions, making choices. It makes me think about, when we’re designing and delivering learning experiences, when we are learning, we’re presumably doing that because we want to achieve something. You can make an argument for learning for the sake of learning, but I think, even then, you’ve got something that you’re trying to achieve, some sort of outcome. And your perspective that comes through a number of times in Make It Meaningful is that the ability to make better decisions is really, broadly speaking, the desired outcome of all learning in a way. Could you say a little bit more about that? Because it makes so much sense, and, when I read that, I was like, “Yes, of course.” But I don’t think I’d heard it quite put that way before. So tell us more about the role of being able to make decisions in learning.
Clark Quinn: [00:18:40] I argue largely that what’s going to make a difference to the individuals you care about, to the organizations that need these people, is not going to be the ability to recite more rote facts. That’s rarely going to be what’s useful. What’s largely going to make a difference in going forward, in our increasingly ambiguous era where rote stuff gets increasingly automated, the ability to make decisions in ambiguous and challenging contexts is going to be what really makes a difference to the success of the organization. And so this arose a little bit as a pushback on Bloom’s taxonomy and other approaches to categorize learning. “Oh, is it this level or this level?” Brenda Sugrue did a pretty good evisceration of that, and she said, “My ultimate thing would be what are the things people need to be able to do? Let’s embed that.” Cathy Moore does that in her action mapping as well. But the key there is what do people need to be able to do?
Clark Quinn: [00:19:38] I argue, again, decisions are going to be what’s most important, and putting them in that situation to make those decisions is going to work. There used to be a belief that you had to ask the rote knowledge questions to ensure that they knew the rote knowledge before you put in the circumstance where they applied that knowledge to the decisions. But, recently, some work…and I’m going to blank on her name. She’s at the Berklee College of Music, but she was a student of Roediger before that. She was a grad student with Roediger and McDaniel or Brown, who wrote the book Make It Stick, which summarized a bunch of good learning research. Her research showed that you could just ask the high-level application questions, and that requires you to retrieve the low-level knowledge sufficiently so that you don’t need the low-level questions. Embedding the core types of performance you need into the environment, scaffolding it, so maybe you have it simplified beforehand, where the numbers all line up really easily, or parts of the problem are solved, that’s all great.
Clark Quinn: [00:20:39] But, eventually, you gradually release more and more roles in the decision-making process until they’re taking it on at the level that you need them to be able to do it at the exit of the learning experience. That’s what makes a difference. When I state what I state about decisions, it’s a way to say what matters is what people need to do. You can argue that, well, executing a process is different than making a discrimination…. Yes, but, at the end of the day, have them making the decisions they need to make. And, if it’s built of component decisions, then Merriënboer’s four-component instructional design talks about the knowledge you need and the complex problems you assign it to. Maybe you have to back up and have some predecessor skills mastered, but those are decisions too. Get those mastered, and then put them together. That way, you get a learning experience that transforms you from where you started to where you end up being. But, still, what’s going to make a difference is at core the ability to make those decisions in the context under ambiguous circumstances. That will be what advanced organizations want and, therefore, what should be embedded in learning.
Jeff Cobb: [00:21:47] Yes, it’s, I guess, one of those elegant ideas. You hear it, and you’re like, “Of course.” But it was a bit of a revelation for me. I think, as I’m now developing, whether it’s a podcast episode or a formal learning experience or even marketing materials, I’m going to be thinking, “What am I trying to help people decide here? How am I facilitating a decision-making process?” And I think that can be very clarifying to think about things that way.
The Potential of AI for Learning
Jeff Cobb: [00:22:21] A little while ago, you mentioned the inevitable, which is artificial intelligence, which, of course, is everywhere now. All sorts of buzz about that. And we don’t necessarily have to talk about artificial intelligence, but I would love to know what has you most excited right now in terms of what’s becoming possible with respect to developing and delivering engaging, effective learning experiences, what already is possible? That may be technology, it may be something else, but what’s on your mind when it comes to possibilities right now?
Clark Quinn: [00:22:54] There are actually several things on my mind right now. One of them is that all the excitement is about generative AI, which is only one form of AI. I’ve been an AI groupie for decades, literally. I happened to be around at the transition from formal symbolic logic to the sub-symbolic models because I was in the lab with Dave Rumelhart. I was in Don Norman’s group within the lab, and other grad students were in Rumelhart’s when he and McClelland came up with the parallel distributed processing that catalyzed the whole machine learning effort that’s now underpinning a lot of what we do. We still have some roles for symbolic learning. Machine learning is mostly discrimination. I don’t mean that in a bad way, although there’s a lot of that too. But I mean discriminating “Is it a this or that?” It’s categorizing. Generative AI generates new things, with some severe caveats around it.
Clark Quinn: [00:23:47] I haven’t been an AI groupie because I think it’s horrible. I’ve been one because I’m excited about the possibilities of modeling the brain and getting useful outputs. But I have a caution there. I think one thing is people are way too negative in one sense, and other people are way too positive, and, of course, the right answer is somewhere in the middle. We have to know what the limitations are, we have to understand how they work, and then we can figure out how to work with them, which goes to…. In my mind, they’re really good partners in thinking, but they should never be trusted completely. You really need a human in the loop that knows enough to be able to go, “That’s garbage.” Narcissist that I am, I tested ChatGPT by asking it what books have I written? It listed four, and three of them were books I’d written, and one of them was a book I’d never written. But it was perfectly confident that those were the four books Clark had written. It didn’t have the other three—or four, actually.
Clark Quinn: [00:24:42] But ChatGPT is based on the Internet. Other ones are based on more rigorous bodies and can be more trustworthy. If you create your own, constraining your sandbox, you can have good faith in it, but, still. Which goes to the broader picture of why my interest in technology. This goes beyond learning, and I think it’s an important message. Technology can be a wonderful augment to our cognition, which is where I am enthused, because it does things well that we don’t and vice versa. If you remember way back to the Foldit app, it was a game to have you fold proteins, and computers would find protein foldings that humans didn’t. But, with that game, humans would find protein foldings that a computer wouldn’t. And, together, we got more than either one alone. If you look at chess-playing, the best competitions are with a computer and a human partner, and the best teams are not the best chess expert nor the best program, but the ones that know how to work together best.
Clark Quinn: [00:25:45] There are times when we shouldn’t try and put it in the head. One of the messages, beyond learning experience, why I talk about cognitive science as well, is we have cognitive gaps in our architectures. Some of that’s knowledge and skills, and we can develop those through courses. But there are other times when it doesn’t make sense. If we’re not seeing it very frequently, any learning we do will be atrophied by the time we come to this situation. We’re far better off providing information in the world, like checklists and step-by-step guides, and, in many cases, those are better and cheaper solutions than putting it in the head. So, while I’m enthusiastic about learning design, I’m also enthusiastic about a good performance consulting up front to decide should it be in the head? And then let’s do it right and make it engaging. Or should it be in the world? And when can we use technology? Increasingly, we can have interactive technology that asks us some information about the context and then can gives us better recommendations and asks us further questions. So we have a dialog with the computer to better solve it, using its strengths and our strengths, than either one alone.
An Approach to Personal Learning and Development
Jeff Cobb: [00:26:49] As we’re wrapping up the conversation, one thing we always like to ask our guests about is their own approaches to lifelong learning. So I’d love to know what are some of your practices right now for your ongoing learning and development?
Clark Quinn: [00:27:06] I like Harold Jarche’s personal knowledge management approach, where he talks about seek—that’s either when you do a search and also the feeds you set up that are tuned to your interest and continually maintaining and updating those as part of the process. And then sensing is making sense of what’s come in. I have two important ways of that. One is that re-representing it, processing it. I love a diagram. I think diagrams are really useful ways to understand what’s happening, the causal relationships between elements, to understand how the world works—basically, mental models. The other way is to experiment, to test it out, to try things. And then the final component of his is share. Seek the information, sense (make sense of it), and share. So I blog. I write books. These are ways that I put it out there. Why do I do that? Because the feedback I get helps fine-tune that. People will comment and go, “What about this?” “Oh, you’re right.” Or I say, “Here’s why I think differently.” And they go, “Oh, okay.” We’re learning together. So those are my practices—diagraming, experimenting, and sharing—but also figuring out what to track, who to track, what tools to play.
Clark Quinn: [00:28:25] I used to be on Twitter. I’m off that now. I’m on Mastodon or Bluesky, and I haven’t yet converged on any of the ones, so I’m continuing to explore them both. Neither one has that interpersonal relationship I had with other folks, so we’ve got some other channels. We’ve got some Discord. We’ve got some Facebook. We’ve got stuff. And I still go to events and interact with people there. I have a wee bit of a problem with that. I’m an introvert. So, if you ever see me at an event, come up and talk to me because I’m too shy to. I’m not aloof. I’m shy. That’s the problem. But those are the practices. Apply what we know. Metalearning, I think, is really important—that was a chapter in my Learning Science book. Our learning to learn matters as well. And, too often, we don’t know. People believe still that highlighting matters or that learning styles are real, and there’s a bunch of stuff that we can understand better—how human learning happens—and apply it to ourselves as well as to those we design for.
Celisa Steele: [00:29:28] Clark Quinn is executive director of Quinnovation, chief learning strategist for Upside Learning, co-director of the Learning Development Accelerator, and science advisor to elevator9. He’s also the author of many books, including Make It Meaningful: Taking Learning Design from Instructional to Transformational and Millennials, Goldfish & Other Training Misconceptions: Debunking Learning Myths and Superstitions.
To make sure you don’t miss new episodes, we encourage you to subscribe via RSS, Apple Podcasts, Spotify, Stitcher Radio, iHeartRadio, PodBean, or any podcatcher service you may use (e.g., Overcast). Subscribing also gives us some data on the impact of the podcast.
We’d also be grateful if you would take a minute to rate us on Apple Podcasts or wherever you listen. We personally appreciate reviews and ratings, and they help us show up when people search for content on leading a learning business.
Finally, consider following us and sharing the good word about Leading Learning. You can find us on X (formerly Twitter), Facebook, and LinkedIn.
Related Resources
- Debunking Learning Myths with Clark Quinn
- Design Learning for Behavior Change with Julie Dirksen
- Learner Surveys and Learning Effectiveness with Will Thalheimer
- On Learning Well: A Practical Look at Metalearning for Learning Businesses
- The Truth in Learning, Leadership, and Motivation with Matthew Richter
- Personal Knowledge Mastery with Harold Jarche
Leave a Reply